How the Spotify algorithms work
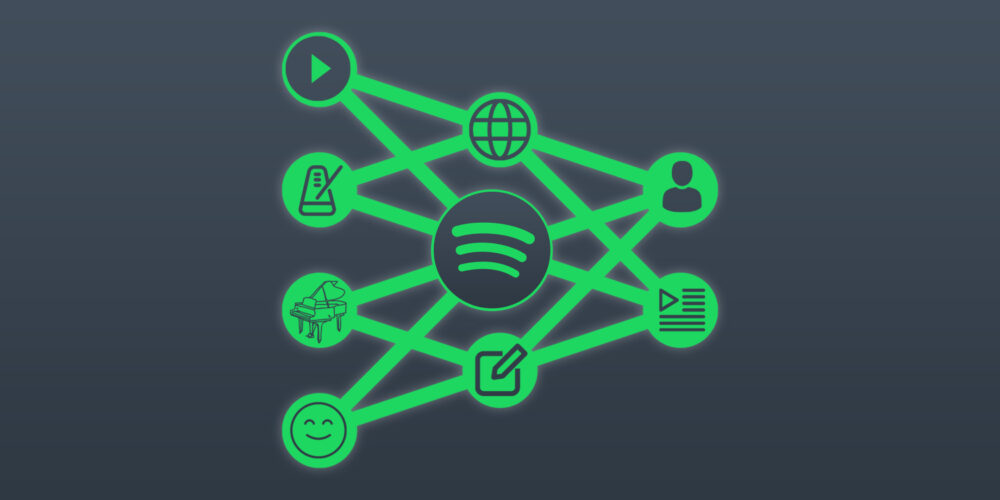
- How detailed Spotify analyzes each song
- How Spotify detects connections between songs
- How the listening behavior of users is x-rayed
- How the recommendations work
As Spotify recently revealed on their “Made to be Found” page, a third of all artist discoveries occur through algorithms. Understanding how these Spotify algorithms work is therefore vital to increase your reach as well as the amount of your streams. Although Spotify obviously keeps the workings of their algorithms secret, there are still quite a few things that are known and many that can be at least surmised. We’ve put it all together for you.
The algorithms pursue the simple goal of keeping the user on the platform longer by serving as an intermediary between the user and the artist – ideally with the artists that the user wants to listen to. When Spotify analyses a song, it does so in two different ways:
- Content: Here, the track and its content is analyzed
- Connection: Here, a connection is made between the track and other songs, based on the user behavior
These two components are what allow Spotify to correctly place and categorize a song directly after it’s uploaded.
Content
A majority of the information is contributed by the artists themselves, when they pitch the song and list all the relevant details. It’s therefore all the more important to take time with the pitch and to fill everything out as accurately as possible. This includes basic information about the song (title, genre, etc.), as well as mood, style, language, and instruments used. But of course, this is not the only data that Spotify collects on a song.
As soon as a new song is uploaded, it goes through the audio analysis process. How that works exactly is probably one of Spotify’s best kept secret. The audio analysis detects whether or not a song has vocals, whether you can dance to it, how high the energy level is, and also whether a track has a more optimistic sound or carries a more somber vibe. But this is just the basis for the analysis. The system goes a lot deeper and really, analyzes the entire structure of the song. At the end, the algorithm knows everything it needs to know about the characteristics of the track.
But not only the entire production is analyzed in detail, the content, too, is carefully dissected. On the one hand, the song lyrics are x-rayed to place the theme and the mood of the song. At the same time, the system scours the internet to find articles about the song on blogs and in the media and see how journalists describe it. And finally, Spotify makes use of the millions of user-generated playlists. If a song is included in a lot of playlists containing the word “sad” in the title, they can safely assume that it’s a more contemplative track.
So, Spotify now knows how the song sounds, what topics it revolves around, and how it is received by the outside world. That’s already a lot of information, but it only makes up half of the track analysis.
Connection
On top of the content, they also consider all the connections attached to it. Just because Spotify knows exactly how a track sounds and what it’s about, it can’t entirely surmise how users interact with it. That’s why this second step is crucial for recommendations.
The general assumption about how recommendations are made on Spotify goes something along these lines: If user A has listened to song X, Y, and Z, and user B likes song X and Y, then user B will want to listen to song Z, as well. But this is an extremely error-prone method, and experts assume that Spotify does not use it anymore, or if they do, then only in a very limited way. Rather, they pay attention to “organizational factors”, meaning, for instance, that two songs are probably similar if a user puts them in the same playlist.
To put it simply, it’s quite possible that a user will listen to hardcore trap music as well as a gentle soul ballad. But the likelihood of them putting these two songs in the same playlist is much lower. The fact that most users give their playlists names, revealing something about the contents, helps Spotify even further. Spotify apparently analyzes 700 million user-generated playlists, so only a fraction of all the playlists made by their consumers. They focus primarily on those that let give the impression that a lot of time and effort went into them.
By analyzing the contents and the connections, Spotify now knows what tracks are similar and therefore have the one side of intermediation down. The other side is, of course, the listeners on the platform.
Analysis of listening behavior
The analysis of users’ listening activity is slightly less complex what they do with the songs, and yet also a little more clever than one may assume. What’s clear is that Spotify thoroughly tracks its user listening activity: which songs are played, which are liked, which are skipped, and so forth and so
But of course, context is taken into account as well, because not every listening session can be assessed the same way. If a user listens to the new releases on their release radar, they might be looking at 100-200 tracks. If they listen to one of these for less than 30 seconds, that doesn’t necessarily mean they’re not interested. Maybe they just want to listen to it later when they’re less busy. If, however, they’re listening to a playlist for studying and skip a song there, it is far more likely that they don’t like the song and don’t want to listen to it anymore.
Generally, user feedback can be divided into two categories:
- Active feedback: Saves, playlist adds, shares, skips, clicks on the artist profile, and artist follows
- Passive feedback: Duration of the listening session, whether or not a track is played all the way through, and whether or not a track is played multiple times
Active feedback is clearly the more important one. Since music is often played in the background, a song played all the way from start to finish doesn’t necessarily mean that the user is in love with it. It could just be that they were taking a quick bathroom break.
Thanks to this feedback, Spotify has a detailed profile of each user. They know which songs and artists are their favorite, which genres, moods, and eras they prefer, and of course, demographic and geographic information are considered, as well. This profile is constantly updated, with the most recent listening activity given more weight. If someone has recently discovered a new genre they like, it will be taken into more consideration than other genres the user has listened to over the years.
Various algorithms at work
So now, Spotify has all the information both about the song and about the listeners and should therefore be able to suggest the right songs in the right moment. This happens in all kinds of ways, be it via “Discover Weekly,” personalized playlists or personalized editorial playlists, suggestions on the home screen, personalized search results, playlist suggestions, or, of course, artist radio and autoplay features. Each of these spaces run on their own algorithms.
The algorithms are therefore capable of showing the user songs that they haven‘t listened to in a while as well as tracks that they don’t know yet, but that they might like due to their listening history. It’s a service that Spotify wants to provide its users, but the main goal is, of course, maximizing user retention and time spent on the platform. As an artist, you may only have limited influence on the algorithms, but with clean metadata and a well-maintained profile, you can at least lay the foundation for them.